|
Keynote Lectures |
|
|
|
|
|
VISIGRAPP is the joint conference that is composed by VISAPP, IMAGAPP, GRAPP and IVAPP. These component conferences are always co-located and held in parallel. Keynote speakers deliver plenary speeches common to all. |
|
|
|
|
|
- Prof. Ali Mohammad-Djafari, Centre National de la Recherche Scientifique (CNRS), France
Title: Regularization and Bayesian Estimation Approach for Inverse Problems in Imaging Systems and Computer Vision
- Prof. Brian A. Barsky, University of California, Berkeley, U.S.A.
Title: Two New Approaches to Depth of Field Postprocessing
- Prof. Pascal Fua, École Polytechnique Fédérale de Lausanne, Switzerland
Title: Modeling Deformable Surfaces from Single Videos
- Dr. Gabriela Csurka, Xerox Research Centre Europe, France
Title: Fisher Kernel Representation of Images and some of its Successful Applications
|
|
|
|
|
|
|
|
|
|
Regularization and Bayesian Estimation Approach
for Inverse Problems in Imaging Systems and Computer Vision
|
|
|
|
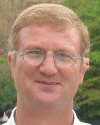 |
|
|
|
|
|
|
|
Brief
Bio
Ali Mohammad-Djafari was born in Iran. He received the B.Sc. degree in electrical engineering from Polytechnique of Teheran in 1975, the diploma degree (M.Sc.) from "Ecole Supérieure d'Electricité (SUPELEC)", Gif sur Yvette, France in 1977, the
"Docteur-Ingénieur" (Ph.D.) degree and "Doctorat d'Etat"in Physics
from "Université Paris Sud 11 (UPS)", Orsay, France, respectively in
1981 and 1987.
He was Associate Professor at UPS for two years (1981-1983). Since 1984, he has a permanent position at "Centre National de la Recherche Scientifique (CNRS)" and works at "Laboratoire des Signaux et Systèmes (L2S)" at "SUPELEC". From 1998 to 2002, he has been at the head of Signal and Image Processing division at this laboratory. In 1997-1998, he has been visiting Associate Professor at University of Notre Dame, Indiana, USA. Presently, he is "Directeur de recherche" and his main scientific interests are in developing new probabilistic methods based on Bayesian inference, Information theory and Maximum entropy approaches for inverse problems in general, and more specifically for signal and image reconstruction and restoration. His recent research projects contain:
Blind Sources Separation (BSS) for multivariate signals (sattelite images, hyperspectral images), Data and Image fusion, Superresolution, X ray Computed Tomography, Microwave imaging and Spatio-temporal Positons Emission Tomography (PET) data and image processing. The main application domain of his interests are Computed Tomography (X rays, PET, SPECT, MRI, Microwave, Ultrasound and Eddy current imaging) either for medical imaging or for Non Destructive Testing (NDT) in industry.
.
Abstract
Inverse problems arise in many imaging and computer vision systems:
image denoising, restoration and reconstruction, super-resolution, fusion or separation. In many imaging applications such as medical imaging or non destructive testing (2D, 3D, 2D+time or 3D+time) describing the problem as an inverse problem is natural, because we have measured data which are related to the unknown quantities through a physical model. In computer vision the problems such as stereo, image fusion, 3D scene reconstruction from shadows or from photographies at different angles, satelite imaging, etc., can also easily be writen as inverse problems. We can also write many problems such as Blind source separation, Compressed sensing, multi or hyperspectral image segmentation as inverse problems and parameter estimation. A common framework for all these problems can be written in an algebraic form and then easily compare the deterministic regularization theory and the probabilistic Bayesian inference frameworks.
Outline:
1. Examples of inverse problems in different area and applications
2. Description in a common mathematical framework
3. Deterministic regularization theory
4. Probabilistic methods
5. Bayesian inference and estimation framework
6. Prior models: from simple separable and markovian to complex and hierarchical markovian models with hidden markovian fields
7. A Computer tomography example where the interest of the Bayesian approach with a Gauss-Markov-Potts prior modeling is shown.
|
|
|
|
|
|
|
|
|
Two New Approaches to Depth of Field Postprocessing |
|
|
|
 |
|
|
|
|
|
|
Brief
Bio
Brian A. Barsky is Professor of Computer Science and Vision Science, and Affiliate Professor of Optometry, at the University of California at Berkeley, USA. He is also a member of the Joint Graduate Group in Bioengineering, an interdisciplinary and inter-campus program, between UC Berkeley and UC San Francisco, and a Fellow of the American Academy of Optometry (F.A.A.O.). Professor Barsky has co-authored technical articles in the broad areas of computer aided geometric design and modeling, interactive three-dimensional computer graphics, visualization in scientific computing, computer aided cornea modeling and visualization, medical imaging, and virtual environments for surgical simulation. He is also a co-author of the book An Introduction to Splines for Use in Computer Graphics and Geometric Modeling, co-editor of the book Making Them Move: Mechanics, Control, and Animation of Articulated Figures, and author of the book Computer Graphics and Geometric Modeling Using Beta-splines. Professor Barsky also held visiting positions in numerous universities of European and Asian countries. He is also a speaker at many international meetings, an editor for technical journal and book series in computer graphics and geometric modelling, and a recipient of an IBM Faculty Development Award and a National Science Foundation Presidential Young Investigator Award. Further information about Professor Barsky can be found at http://www.cs.berkeley.edu/~barsky/biog.html.
Abstract
Depth of field refers to the swath that is imaged in sharp focus through an optics system, such as a camera lens. Control over depth of field is an important artistic tool, which can be used, for example, to emphasize the subject of a photograph. The most efficient algorithms for simulating depth of field are postprocessing methods. Post-processing can be made more efficient by making various approximations. We start with the assumption that the point spread function (PSF) is Gaussian. This assumption introduces structure into the problem which we exploit to achieve speed.
Two methods will be presented. In our first approach, PSFs are spread into a pyramid. By writing larger PSFs to coarser levels of the pyramid, the performance remains constant independent of the size of the PSFs. After spreading all the PSFs, the pyramid is then collapsed to yield the final, blurred image. Our second approach exploits the fact that blurring is a linear operator. The operator is treated as a large tensor which is compressed by finding structure in it. The compressed representation is then used to directly blur the image. Both methods present new perspectives for the problem of efficiently blurring an image.
|
|
|
|
|
|
|
|
|
Modeling Deformable Surfaces from Single Videos |
|
|
|
 |
|
|
|
|
|
|
Brief
Bio
Pascal Fua received an engineering degree from Ecole Polytechnique, Paris, in 1984 and the Ph.D. degree in Computer Science from the University of Orsay in 1989. He joined EPFL (Swiss Federal Institute of Technology) in 1996 where he is now a Professor in the School of Computer and Communication Science. Before that, he worked at SRI International and at INRIA Sophia-Antipolis as a computer scientist. His research interests include shape modeling and motion recovery from images, human body modeling, and optimization-based techniques for image analysis and synthesis. He has (co)authored over 150 publications in refereed journals and conferences. He has been an associate editor of IEEE journal Transactions for Pattern Analysis and Machine Intelligence and has often been a program committee member and area chair of major vision conferences.
Abstract
Without a strong model, 3--D shape recovery of non-rigid surfaces from monocular video sequences is a severely under-constrained problem. Prior models are required to resolve the inherent ambiguities. In our work, we have investigated several approaches to incorporating such priors without making unwarranted assumptions about the physical properties of the surfaces we are dealing with.
In this talk, I will present these approaches and discuss their relative strengths and weaknesses. I will also demonstrate that they can be incorporated into effective algorithms that can capture very complex deformations.
|
|
|
|
|
|
|
|
|
Fisher Kernel Representation of Images and some of its Successful Applications |
|
|
|
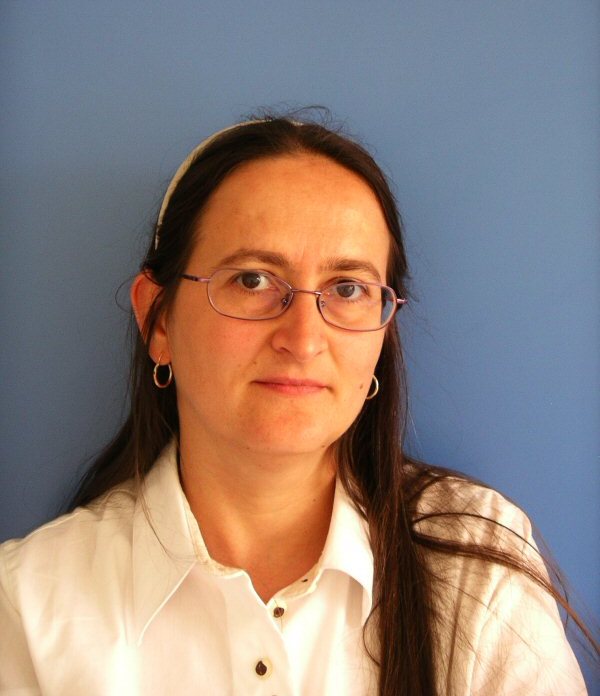 |
|
|
|
|
|
|
Brief
Bio
Gabriela Csurka is a research scientist in the Textual and Visual Pattern Analysis team at Xerox Research Centre Europe (XRCE). She obtained her Ph.D. degree (1996) in Computer Science from University of Nice Sophia - Antipolis. Before joining XRCE in 2002, she worked in fields such as stereo vision and projective reconstruction at INRIA (Sophia Antipolis, Rhone Alpes and IRISA) and image and video watermarking at University of Geneva and Institute Eurécom, Sophia Antipolis. Author of several publications in main journals and international conferences, she is also an active reviewer both for international journals (IJCV, TPAMI, TIP, PATREC, etc) and main computer vision conferences. Her current research interest concerns the exploration of new technologies for image content and aesthetic analysis, mono and cross-modal image categorization and retrieval, semantic based image segmentation.
Abstract
The Fisher Kernel representation (FK) of images can be seen as an extension of the popular bag-of-visual word (BOV) representation. Both of them are based on an intermediate representation, the visual vocabulary built in the feature space. If a probability density function (in our case a Gaussian Mixture Model) is used to model the visual vocabulary, we can compute the gradient of the log likelihood with respect to the parameters of the model to represent an image (Perronnin and Dance, CVPR 2007). The Fisher vector is the concatenation of these partial derivatives and describes in which direction the parameters of the model should be modified to best fit the data.
This representation has the main advantage to give similar or even better classification performance than BOV obtained with supervised visual vocabularies, being at the same time class independent. This latter property allows its usage both in supervised (categorization, semantic image segmentation) and unsupervised tasks (clustering, retrieval).
Outline:
• The bag-of-visual word (BOV) and Fisher Kernel image representation.
• Visual Concept Detection and Image Auto-annotation.
• Semantic Image Segmentation and Intelligent Auto-thumbnailing.
• Cross-modal Image Retrieval and Hybrid Content Generation.
|
|
|
|
|
|
|